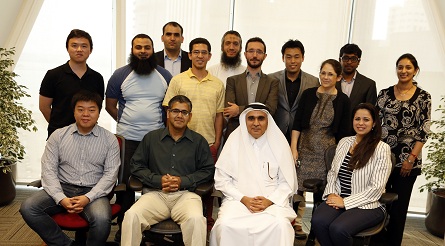
QCRI’s Qatar Center for Artificial intelligence Group research expertise transcends the data science stack - from data extraction and cleaning to analytics, retrieval, inference and prediction. The group expresses its research by publishing in the world’s leading computer science conferences and journals, and through engineering prototype software systems that are being used by researchers and practitioners worldwide. More specifically, the group is working on the following projects:
- RHEEM is the world’s first cross-platform system which seamlessly decouples data science application tasks from their underlying execution platform. Users specify data analytical tasks, and the RHEEM platform will automatically decide on which combination of platforms to execute the tasks on. The RHEEM platform includes ML4ALL, a machine learning suite that is specifically designed for big data.
- The Data Civilizer project, in partnership with MIT, is carrying out research to resolve a core bottleneck facing all data-rich organizations – how to automatically extract knowledge from thousands of heterogeneous data sources that are scattered throughout an enterprise. The technology developed as part of a project is being used by a large pharmaceutical company for data integration and analysis.
- The Kharita map inference algorithm infers road networks from noisy GPS traces in real time. Kharita is being used to automatically update maps in environments witnessing rapid changes and where existing map and navigation applications quickly become out-of-sync with the ground realities.
- Rayyan is a sophisticated and fast-growing text retrieval system for carrying out systematic reviews in the medical domain. Researchers use Rayyan to efficiently evaluate competing claims regarding a medical hypothesis under investigation.
- ASM infers road graphs from satellite imagery is a challenging computer vision task. Prior solutions fall into two categories: (1) pixel-wise segmentation-based approaches, which predict whether each pixel is on a road, and (2) graph-based approaches, which predict the road graph iteratively. We find that these two approaches have complementary strengths while suffering from their own inherent limitations. In this paper, we propose a new method, Sat2Graph, which combines the advantages of the two prior categories into a unified framework. The key idea in Sat2Graph is a novel encoding scheme, graph-tensor encoding (GTE), which encodes the road graph into a tensor representation. GTE makes it possible to train a simple, non-recurrent, supervised model to predict a rich set of features that capture the graph structure directly from an image. We evaluate Sat2Graph using two large datasets. We find that Sat2Graph surpasses prior methods on two widely used metrics, TOPO and APLS. Furthermore, whereas prior work only infers planar road graphs, our approach is capable of inferring stacked roads (e.g., overpasses), and does so robustly.
- QarSUMO is a parallel, congestion-optimized version of the popular SUMO open-source traffic simulator. QarSUMO performs high-level parallelization on top of SUMO, to utilize powerful multi-core servers and enables future extension to multi-node parallel simulation if necessary. The proposed design, while partly sacrificing speedup, makes QarSUMO compatible with future SUMO improvements. We further contribute to such an improvement by modifying the SUMO simulation engine for congestion scenarios where the update computation of consecutive and slow-moving vehicles can be simplified. We evaluate QarSUMO with both real-world and synthetic road network and traffic data, and examine its execution time as well as simulation accuracy relative to the original, sequential SUMO.
The Qatar Center for Artificial intelligence group has also established deep research partnerships with The Boeing Company and Qatar Airways in the areas of predictive maintenance and cargo prediction.